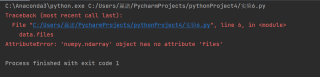
#建立模型
import numpy as np
import tensorflow as tf
#读取数据
data = np.load('mnist.npz')
data.files
train_images, train_labels, test_images, test_labels = data['x_train'], data['y_train'], data['x_test'],
data['y_test']
print(train_images.shape)
print(train_labels.shape)
print(test_images.shape)
print(test_labels.shape)
#交叉熵
target_y = np.array([1, 0, 0, 0, 0, 0, 0, 0, 0, 0])
predicted_y1 = np.array([0.4, 0.5, 0.1, 0, 0, 0, 0, 0, 0, 0])
predicted_y2 = np.array([0.1, 0.2, 0.7, 0, 0, 0, 0, 0, 0, 0])
-np.sum( target_y * np.log(predicted_y1+0.0000001))
-np.sum( target_y * np.log(predicted_y2+0.0000001))
#搭建网络结构
model = tf.keras.models.Sequential()
model.add(tf.keras.layers.Flatten(input_shape=(28,28)))
model.add(tf.keras.layers.Dense(128, activation = 'relu'))
model.add(tf.keras.layers.Dense(10, activation = 'softmax'))
#编译模型
model.compile(optimizer = 'adam', loss = 'sparse_categorical_crossentropy', metrics = ['accuracy'])
#训练
model.fit(train_images, train_labels, verbose = 1, epochs = 20, validation_data = (test_images,
test_labels))
#模型保存
model.save('model_mnist.h5')
#用模型进行预测
import tensorflow as tf
import matplotlib.pyplot as plt
model = tf.keras.models.load_model('model_mnist.h5')
model.summary()
for i in range(30):
image = plt.imread('testimages/' + str(i) + '.jpg')
image_new = image.reshape([1, 28, 28])
result = model.predict(image_new)[0].argmax()
print('The', i + 1, 'th picture shows:', result)